The human labor behind AI chatbots and other smart tools
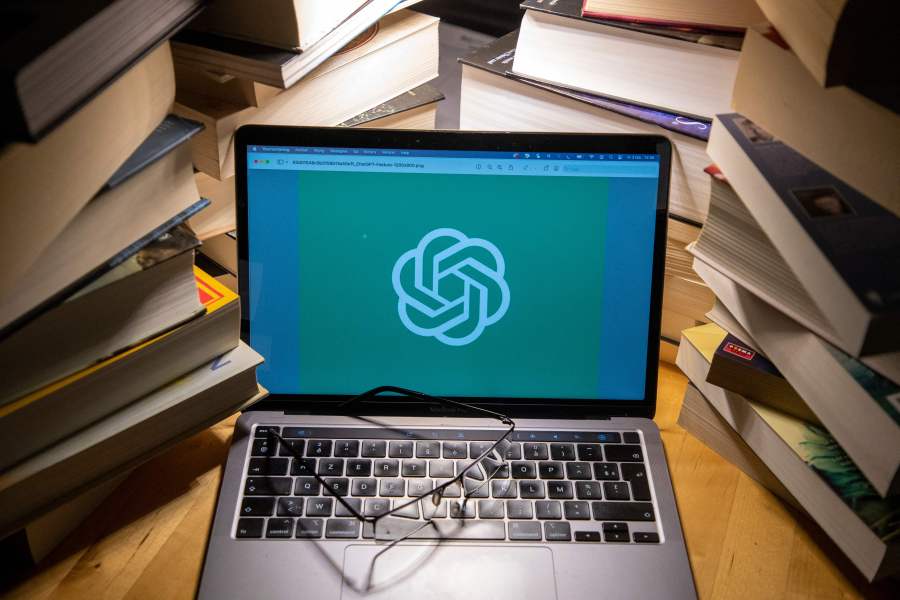
Every week it seems the world is stunned by another advance in artificial intelligence, including text-to-image generators like DALL-E and the latest chatbot, GPT-4.
What makes these tools impressive is the enormous amount of data they’re trained on, specifically the millions of images and words on the internet.
But the process of machine learning relies on a lot of human data labelers.
Marketplace’s Meghan McCarty Carino spoke to Sarah T. Roberts, a professor of information studies and director of the Center for Critical Internet Inquiry at UCLA, about how this work is often overlooked. The following is an edited transcript of their conversation.
Sarah T. Roberts: In the case of something like ChatGPT and the engines that it’s using, it’s really going out and pretty much data mining massive portions of the internet. Now, we all know that the internet is filled with the best information and the greatest stuff all the time, right? So what’s required for something like that is to have human beings with their special ability of discernment and good judgement, and sometimes visceral reactions to material — and in the case of ChatGPT, to cull material out, material that users, or more importantly, companies, would not want inside of their products as a potential output. And so that means these data labelers, much like content moderators, spend their days working on some of the worst stuff that we can imagine. And in this case, they’re trying to build models to cull that out automatically. But it always starts and ends with human engagement.
Meghan McCarty Carino: What do we know about the people who are doing this really key work of data labelling?
Roberts: So taking a page from the content moderation industry, much of this work is outsourced to third-party companies that provide large labor pools. Often these data labelers are at great remove from where we might imagine the work of engineering these products goes on. They might be in other parts of the world. There was a great article by Billy Perrigo in Time magazine in January of 2023, about a place in Kenya that was doing data labelling. It was a really hard, upsetting job, and folks were being paid at most $2 an hour to be confronted with that material. Unfortunately, this is an industry that is reliant upon human intervention and human discernment, but once again, takes it for granted and pays very little and puts people in harm’s way.
McCarty Carino: Right, very similar to, you know, what we’ve learned about content moderation, which, as you said, happens in a similar sort of outsourced way where these people are sort of the front lines of everything that we don’t want showing up in our end product, and it runs through these workers.
Roberts: Yeah, that’s right. And for years, I’ve been listening to industry figures and other pundits tell me that my concern about the welfare of content moderation workers was appropriate, but it was finite, and that in just a few years, AI technologies would be such that we could eliminate that work. In fact, what’s happening is just the opposite. We are expanding, greatly expanding at an exponential pace, the number of people who are doing work like this. I think of data labelling frankly as content moderation before the fact, both in practice, but also in the material conditions of the work.
McCarty Carino: When we think about how these technologies are often described, or characterized by the companies that put them out or, you know, in the press, I mean, what is important to keep in mind as we think about this type of labor and its relationship to those products?
Roberts: I think what we have to remember is that AI is artificial intelligence leaning heavily on the artificial. And what it’s doing at best is imitating human discernment, thinking and processes, but it is only as good as the material that goes into it. You know, there’s an old adage in programming, “garbage in, garbage out,” that goes maybe even more so for applications like these AI tools that we’ve been discussing. Emily Bender and her colleagues wrote a great paper called stochastic parrots, which is how she and her colleagues describe what ChatGPT is actually doing. And for those who aren’t familiar with that term, basically, what she’s saying is that you can use ChatGPT, it’s incredible, I’ve used it as well. But you have to keep in mind that what you’re seeing as its output is at best mimicking humans in the same way that a parrot might copy our pattern of speech, a series of words or phrases, even our inflection, but really has no cognitive ability to necessarily understand what those things mean.
And in fact, I would give a parrot a better chance of having that kind of cognition than I would have machine. So in a way, I’ve been thinking about ChatGPT and other tools like it really as vanity machines. Just as an example, I requested it to generate an annotated bibliography for me the other day in my own field. I picked something that I thought I would have some expertise in in order to evaluate the output. And it gave me about 10 answers. The first one it gave me was something I would have chosen as well, a book by a colleague. Perfect response. And then it started producing a bunch of new papers and books in my area of study that I’d never heard of. And I really thought, “Wow, have I really been underwater that much during COVID? Like, all this stuff is coming out and I’m missing it?” Turns out, those were fake citations, fake authors, fake books on legitimate presses, fake papers, but using legitimate journal titles with even page numbers given. Imagine if I hadn’t had the expertise to know that those were bogus. That’s just one example of the way that this stochastic parrot or this mimicry might reproduce. And, of course, to be fair, I didn’t ask it to give me real citations or truthful information. It gave me its best guess at what an annotated bibliography would look like in my field. But none of it was real.
McCarty Carino: What gets lost when tools like this are thought of as these sort of genius technological achievements without considering all of the human labor that went into them?
Roberts: They could have really chosen any model. They could have decided, you know, an infinite number of possibilities of how to set up that work and how to treat those workers. And I think it says something about tech companies. The actual intelligence that they are mining, the very essence of what makes these tools appear to have this human element — in other words, mimicking the humans that work on the labeling, work on the moderation, work on these inputs — are erased from the process. And I think the erasure of the humanity that goes into these tools is to all of our detriment if for no other reason then we can’t really fully appreciate the labor that goes into creating them or the limits of the tools and how they should be applied.
Related links: More insight from Meghan McCarty Carino
A report from Grand View Research valued the global data collection and labeling market over $2.2 billion in 2022. It’s a huge sector.
And it’s important to understand it’s not just this new generative AI that requires this kind of work. For example, my colleague Jennifer Pak reported a couple years ago on a data labeling center in China that contracts with big companies like Baidu and Alibaba.
One of the workers Jennifer spoke to said he was making twice the average salary in his local province, roughly $11,000 a year, plus commission.
The operation had workers labeling street data for an autonomous vehicle project — basically, “That’s a bike, that’s a pedestrian, that’s a baby stroller.”
The same type of labor is used to label faces to train facial recognition software or to help robot vacuums navigate their way around your home.
Earlier this year, we spoke to MIT Technology Review reporter Eileen Guo about her story on how sensitive personal images taken by robot vacuums inside people’s homes ended up online.
It’s a winding path, but it runs through a group of outsourced data labelers in Venezuela that iRobot contracted.
The future of this podcast starts with you.
Every day, the “Marketplace Tech” team demystifies the digital economy with stories that explore more than just Big Tech. We’re committed to covering topics that matter to you and the world around us, diving deep into how technology intersects with climate change, inequity, and disinformation.
As part of a nonprofit newsroom, we’re counting on listeners like you to keep this public service paywall-free and available to all.
Support “Marketplace Tech” in any amount today and become a partner in our mission.